Data is now the soul of every organization. Placing data at the center of your business strategy gives you a competitive advantage in today’s digital age. According to Gartner, D&A is shifting to become a core business function, rather than it being a secondary activity done by IT to support business outcomes. Business leaders now think of D&A as a key business capability to drive business results.
You must now concentrate your digital transformation efforts on adopting new data-driven technologies and processes for more valuable insights from data so that you can use them to address future needs.
The Need for a Robust Data Architecture
Data management architecture defines the way organizations gather, store, protect, organize, integrate, and utilize data. A robust data management architecture defines every element of data and makes data available easily with the right governance and speed. A bad data management architecture, on the other hand, results in inconsistent datasets, incompatible data silos, and data quality issues, rendering data useless or limiting an organization’s ability to perform data analytics, data warehousing (DW), and business intelligence (BI) activities at scale, particularly with Big Data.
The Journey and the Challenges You Will Likely Encounter
Most organizations start their journey with a centralized data team and a monolithic data management architecture like a data lake, in which all data activities are performed from and to a single, centralized data platform. While a monolithic data architecture is simple to set up and can manage small-scale data analytics and storage without sacrificing speed, it quickly becomes overwhelming. Furthermore, as data volume and demand grow, the central data management team becomes a bottleneck. Consequently, there is a longer delay to insight and a loss of opportunity.
To enhance and improve your capability to extract value out of data, you should embrace a new approach, like data mesh, for handling data at scale. Although previous technical advances addressed data volume, data processing and storages, they could not handle scale in additional dimensions such as the increase of data sources, changes in the data landscape, speed of reaction to change, and variety of data consumers and use cases. These aspects are addressed by a data mesh architecture, which promotes a novel logical perspective of organizational structures and technological design.
What is Data Mesh?
To harness the real potential of data, Data Mesh uses current software engineering techniques and lessons learned from developing resilient, internet-scale applications. As described by Zhamak Dehghani, Data mesh is a decentralized socio-technical approach to managing analytical data at scale. It is a method to reconcile and hopefully solve issues that have troubled initial data designs, which are often hampered by data standards issues between data consumers and producers. The data mesh pushes us toward domain-driven architecture and empowered, agile, and smaller multi-function teams. It combines the most acceptable data management methods while maintaining a data-as-a-product perspective, self-service user access, domain knowledge, and governance.
Some principles must be followed to achieve an effective data mesh. These principles demand maturity of the organization’s culture and data management.
- Domain-oriented data ownership and architecture: As domain ownership has changed in modern digital organizations, where product teams are aligned with the business domain. A data mesh approach empowers product teams to own, govern, and share the data they generate in a regulated and consistent manner. This method combines data understanding with data delivery to accelerate value delivery.
- Data as a product: Rather than considering data as an asset to be accumulated by establishing responsibility with the data product owner, a shift to product thinking for data allows higher data quality. Data products should be coherent and self-contained.
- Self-serve data infrastructure: The goal of building a self-serve infrastructure is to give tools and user-friendly interfaces so that developers can create analytical data products faster and better. This method assures compliance and security while also reducing the time it takes to gain insights from data.
- Federated computational governance: Traditional data platforms are prone to centralized data governance by default. A federated computational governance architecture is required for data mesh, which preserves global controls while improving local flexibility. The platform manages semantic standards, security policies, and compliance from a central location, while the responsibility for compliance is delegated to data product owners.
Benefits of Adopting a Data Mesh Design
Organizations benefit from adopting an effective data mesh design for several reasons, including:
- Decentralized data operations and self-serve infrastructure enable teams to be more flexible and operate independently, improving time-to-market and lowering IT backlog
- Global data governance rules encourage teams to generate and distribute high-quality data in a standardized, easy-to-access manner
- Data mesh empowers domain experts and product owners to manage data while also encouraging greater collaboration between business and IT teams
- Data mesh’s self-serve data architecture takes care of complexity like identity administration, data storage, and monitoring, allowing teams to concentrate on providing data more quickly
At the same time, data-driven improvements like these may help cut operational expenses, drastically reduce lead times, and allow business domains to prioritize and make timely choices that are relevant to them. Also, it makes data accessible across the business while also allowing for technical flexibility.
Is Data Mesh Right For You?
It is essential to keep in mind that a data mesh is one of many data architecture approaches. One must first determine, if your objective and goals are compatible with this new paradigm or whether a different one would be more appropriate for your organization. Ask yourself these quick questions:
- What is the level of collaboration between your data engineers, data owners, and data consumers?
- Is it difficult for these parties to communicate with one another?
- Is your data engineers’ lack of business domain expertise a major productivity bottleneck?
- Do your data users have productivity challenges as a result of this?
- Are you dealing with unavoidable domain-specific business variations in data across business units?
If you responded yes to these questions, particularly the last one, a data mesh may be a good match for your needs. If that is the case, you should begin by gaining executive backing, establishing a budget, identifying domains, and assembling your data mesh team.
Are you still wondering whether or not data mesh is the right choice for you?
Our data specialists can assist you in defining your data strategy, reach out to our data architecture experts.
Recent Blogs
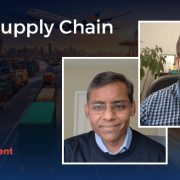
From Data to Decisions: The Evolving Role of Data Science & AI in Supply Chain
March 20, 2025
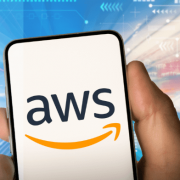
Transforming Enterprise AI with AWS Bedrock and Nova
March 11, 2025
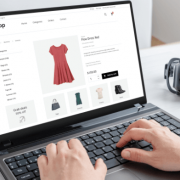
Boosting E-commerce Conversions with Generative AI-Powered Product Descriptions
March 4, 2025
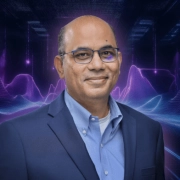
Navigating the AI Landscape: Strategies for Effective Implementation in 2025
March 4, 2025