As technology continually evolves at an impressive rate, artificial intelligence (AI) is becoming an essential part of various industries, including medicine, finance, education, and economics. However, as AI becomes more prevalent, it is absolutely essential that we turn our focus to the security aspect of these systems. The exponential increase in reliance on AI necessitates a framework with unassailable security to safeguard our data and protect our resources.
Importance of Data Security in AI Systems
In the AI realm, data is the backbone of all operations; it fuels the algorithms, drives predictive capabilities, and allows for advanced problem-solving. As the saying goes, “garbage in, garbage out”: without high-quality, accurate data, an AI system is useless at best and dangerous at worst. Therefore, ensuring data security is not just an option or an add-on but a fundamental requirement.
Securing data in AI systems can be challenging because data is continuously flowing – data-in-transit, data-at-rest, and data-in-use, each requiring unique security considerations. Regardless, protecting against cyber threats, leaks, unauthorized access, and tampering should always be prioritized. A breach can not only lead to data loss but also produce incorrect AI outputs, compromising processes and decisions based on those outputs.
Ensuring Access Control and Authentication
The question of ‘who has access’ to data in AI systems is a significant determinant of its overall security posture. Ensuring access control and authentication mechanisms are a part of the integrated security measures in an AI framework.
Having an efficient access control strategy denies unauthorized users access to certain realms of data in the AI system, hence minimizing the risk of a potential data breach. This strategy involves categorizing users and defining their access rights and privileges, giving only the necessary level of access to each category to perform their tasks.
Authentication, on the other hand, is the process of confirming that users are who they claim to be. This process helps keep the AI system secure by preventing fraudulent access or manipulations leading to data breaches. Employing multi-factor authentication (MFA) adds an additional layer of security by requiring users to provide two or more verification factors to gain access.
Security of Data Storage
Last but equally important in the secure AI framework is the security of data storage. Where and how we store our data ultimately determines its security, accessibility, and protection against potential threats.
Data could be stored in one of the three forms, on-premises storage, cloud storage, or hybrid storage. Each of these has its own pros and cons, so an organization must make informed decisions based on their individual requirements and constraints.
Regardless of the storage choice, best practices require data encryption both at rest and during transmission. Encryption renders data unreadable, only allowing access to those possessing a correct encryption key. Regular backups should also be established as a part of a disaster recovery plan.
In addition, it’s crucial to work with trustworthy service providers when using cloud storage solutions. You must ensure adherence to industry-standard protocols and regulatory compliances, such as HIPAA for health information or PCI DSS for credit card data.
Security’s Vital Role in Responsible AI
As we navigate through the intricate world of AI, ensuring the security of our AI systems is paramount. By understanding the importance of data security, implementing robust access control, and placing a high priority on secure data storage, we can greatly mitigate potential security risks.
After all, a responsible AI framework is not only about achieving AI’s full potential. It encompasses gaining the trust in the system’s reliability and accuracy. And without security, there can be no trust. Hence, integrating these components into an AI framework is not just a necessity but an absolute responsibility.
Recent Blogs
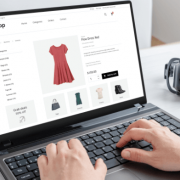
Boosting E-commerce Conversions with Generative AI-Powered Product Descriptions
March 4, 2025
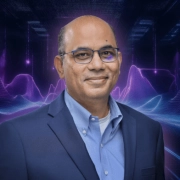
Navigating the AI Landscape: Strategies for Effective Implementation in 2025
March 4, 2025
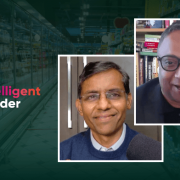
BI in the Age of Generative AI: What’s Changing and What’s Next?
March 3, 2025
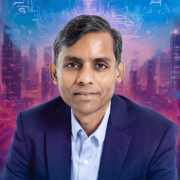
Innovating with Data and AI: Key Industry Impacts in 2025
February 24, 2025