Yes, it really is a million-dollar question. And not just metaphorically—many enterprises are already investing that much or more as they navigate the rapidly evolving world of Large Language Models (LLMs), trying to decide between consuming third-party models through APIs or hosting them internally.
At Infocepts, we’ve been deep in this debate too. But rather than just theorizing, we rolled up our sleeves and got hands-on. Over the past few months, we’ve been rigorously experimenting with self-hosted, fine-tuned LLMs to determine what works best in real-world enterprise scenarios. For simplicity, we call these Domain Language Models (DLMs) or Business Language Models (BLMs)—customized models enriched with organization-specific data and context.
Here’s what we learned—and why this decision could make or break your long-term AI strategy.
Many organizations jump straight into consuming LLMs via APIs from popular providers like OpenAI or Anthropic. These models offer quick wins and ease of use—perfect for experimenting or powering generic use cases like summarization, simple Q&A, or non-contextual content generation.
But when it comes to solving business-specific challenges—think: creating tailored data pipelines, automating domain-heavy processes, or answering context-rich queries—these general-purpose models begin to fall short.
That’s where our experimentation came in. We asked: What if we fine-tuned an open-source base model with real business data? Could it deliver better outcomes at a lower cost, while preserving our data privacy? Spoiler alert: It did!
We started with a base Llama 2.0 7B model—one of the most promising open-source LLMs available. Using Hugging Face’s AutoTrain and Transformers Library, we fine-tuned it on a specific set of data engineering use cases, supported by structured and labeled data from real projects.
We hosted the model using a modest NVIDIA A10G GPU, costing about $1.21 per hour. With this setup, we were able to process 0.5 to 1 million tokens per day, which translated to a monthly cost of around $1000–$1500.
Compare that to the cost of running those same use cases on GPT-4 or Claude via APIs, and the savings become evident fast—especially as usage scales.
To measure impact, we ran a comparison between our fine-tuned BLM and third-party LLMs (GPT-4.5 and Claude 3.7). We used the same set of prompts to generate data transformation queries and build automated data pipelines.
The results were striking:
- Our self-hosted BLM produced more accurate and context-aware responses on the first attempt.
- The API-based models, while powerful, often assumed incorrect business context—leading to hallucinations and wrong outputs.
- Accuracy rate for the self-hosted model was 85–90%, compared to ~70% for the API-based ones.
This difference may seem small at first glance, but in real-world enterprise applications, every error leads to rework, reduced productivity, and lost trust in AI. The ability to generate the right output the first time becomes a game-changer.
By combining lightweight, business-specific LLMs with agentic architectures or Autonomous Process Agents (APA), we believe enterprises can fundamentally transform the way they work. Some game-changing possibilities include:
- Executing end-to-end data engineering sprints in hours instead of weeks
- Accelerating technology migrations, with code and logic translation done in days
- Generating deep, business-aware insights from historical and real-time data
- Enabling sales teams to build personalized pitches instantly, based on what’s worked (and what hasn’t)
- Proactively mitigating risk, using contextual intelligence baked into the model
These are just a few examples. The real impact? You’re not just using AI—you’re shaping it to reflect the DNA of your business.
Another significant consideration is data security and privacy. In our discussions with clients across healthcare, finance, and retail domains, this has emerged as a consistent theme. Nearly 60% of organizations are hesitant—or even delaying—AI adoption due to fears of exposing sensitive business data to third-party APIs.
With self-hosted BLMs, you keep full control. Your data stays within your infrastructure, compliant with your governance and security policies. There’s no worry about what’s being logged, where your prompts go, or how the outputs are being used.
This control is not just a “nice-to-have”—for regulated industries, it’s a must-have.
Of course, self-hosting isn’t all sunshine and savings. It comes with its own learning curve:
Infrastructure Setup: You need the right hardware, cloud environment, and DevOps maturity.
- Expertise Gap: Fine-tuning and maintaining LLMs require niche skills—ML engineers, data scientists, and MLOps talent.
- Ongoing Maintenance: Like any other system, models need versioning, retraining, and monitoring.
That said, once the foundation is in place, the ROI becomes very clear. The upfront cost is quickly recovered through gains in efficiency, quality, and reduced dependence on expensive API calls.
At Infocepts, we believe the future of AI in enterprises lies in hybrid architectures—where API models offer rapid prototyping and access to general intelligence, while self-hosted BLMs deliver contextual depth and cost control.
We’re continuing our work to expand this framework and test BLMs across more domains—from supply chain to customer service to financial reporting. Each use case teaches us more, and we’re refining a blueprint that others can adopt.
We’ll be sharing more technical breakdowns, architectural patterns, and case studies soon. Stay tuned!
Thinking of building your own BLM or DLM? Talk to Infocepts to design a high-impact, sustainable LLM strategy tailored to your business.
Recent Blogs
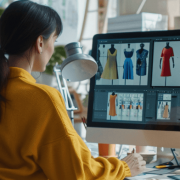
Fashion Retail in the Age of AI: Redefining Design and Customer Experience with Data & Intelligence
April 11, 2025
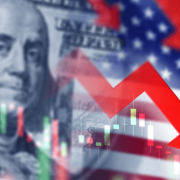
Navigating the Tariff Shock: How AI and Advanced Analytics Fuel Supply Chain Resilience
April 7, 2025
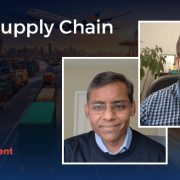
From Data to Decisions: The Evolving Role of Data Science & AI in Supply Chain
March 20, 2025
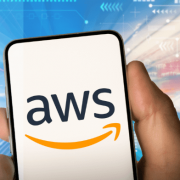
Transforming Enterprise AI with AWS Bedrock and Nova
March 11, 2025